What is statistical significance?
Statistical significance is the observed difference between groups or variables that is unlikely to have occurred by chance.
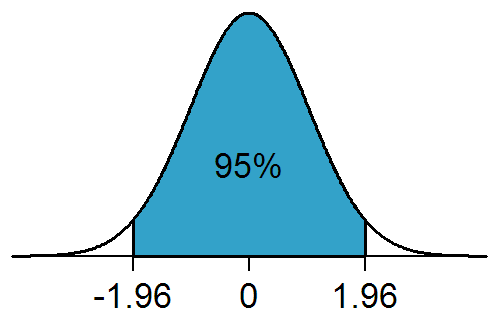
Statistical significance is the observed difference between groups or variables that is unlikely to have occurred by chance.
Statistical significance is a statistics term to determine whether an observed difference or relationship between two or more groups or variables is unlikely to have occurred by chance. It is the probability of obtaining a result as extreme or more extreme than the observed result, assuming that there is no real difference or relationship between the groups or variables being compared.
Statistical significance is an important concept in research because it helps researchers to determine whether their results are meaningful or not. If a result is not statistically significant, it means that there is a high probability that the result could have occurred by chance alone. In this case, the result may not be meaningful and further research may be needed.
There are a number of different ways to calculate statistical significance. The most common way is to use the p-value. The p-value is a number that represents the probability of obtaining the observed result if the null hypothesis were true. The null hypothesis is the hypothesis that there is no difference between the groups being studied.
Here are some examples of statistical significance:
In each of these examples, statistical significance provides evidence that there is a real difference or relationship between the variables being compared and that this difference or relationship is not due to chance alone.
Here are a few tips for understanding statistical significance:
Statistical significance is a measure of the likelihood that the observed difference or relationship is not due to random chance but is instead due to some underlying cause or effect. This measure is often expressed as a p-value, which is the probability of obtaining a result as extreme or more extreme than the observed result, assuming that there is no real difference or relationship between the groups or variables being compared.
If the p-value is less than a predetermined level of significance (usually 0.05 or 0.01), then the result is considered statistically significant, which means that there is strong evidence that the observed difference or relationship is not due to chance alone. If the p-value is greater than the level of significance, then the result is considered not statistically significant, which means that there is insufficient evidence to conclude that the observed difference or relationship is not due to chance alone.
Statistical significance is widely used in many fields, including science, social science, medicine, engineering, and economics, to make decisions and draw conclusions based on data analysis. Here are some of the ways statistical significance is used:
Overall, statistical significance is a powerful tool that enables researchers to draw valid conclusions from their data and make evidence-based decisions. However, it is important to keep in mind that statistical significance alone does not necessarily imply practical significance or real-world importance.
Try running your statistical significance tests on survey data with mTab for free.
Make smarter decisions faster with the world's #1 Insight Management System.